Pentester.com ETL Pipeline Development
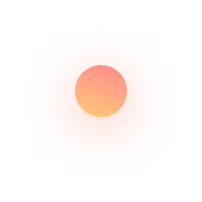
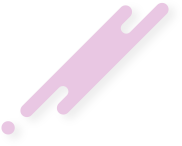
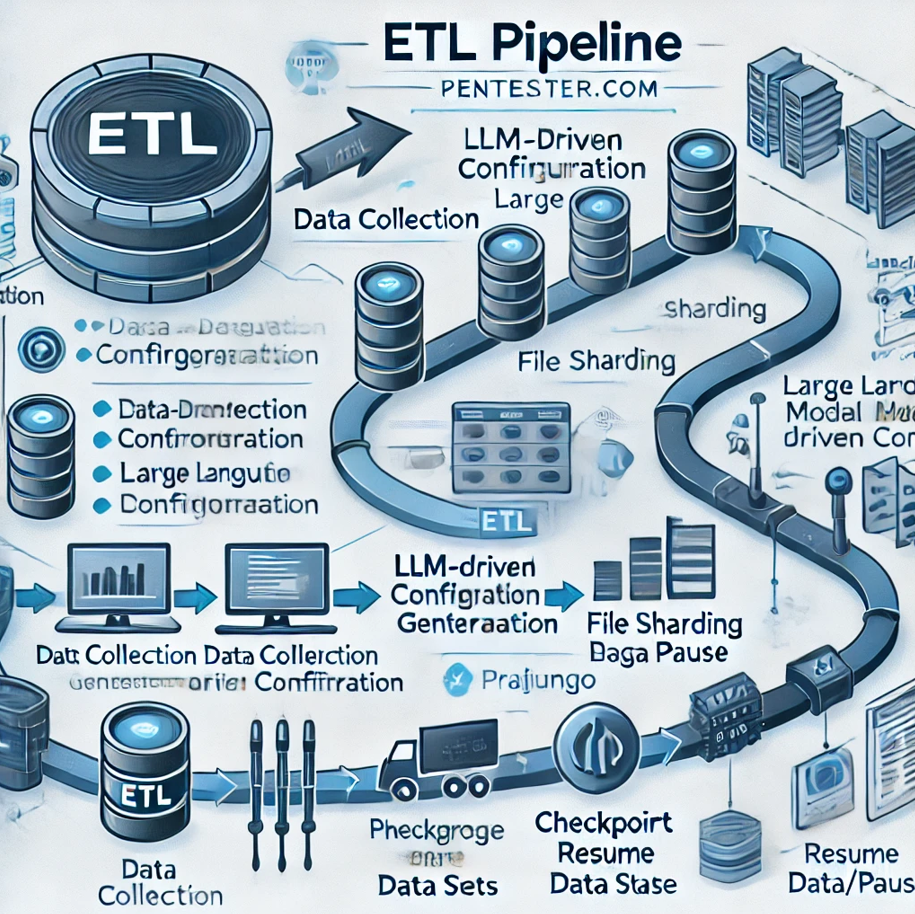
About the Pentester ETL Pipeline
Designed an ETL pipeline for Pentester.com to handle the extraction, transformation, and storage of large-scale profile information from raw data sources. With 8TB of data to process, requiring up to a month to complete, I implemented advanced features like dynamic configuration generation, file sharding, and checkpoint-based resume/pause functionality to maximize efficiency and data integrity.
Challenges
Automate data extraction and parsing with minimal manual intervention.
Implement checkpoint-based processing to enable resumption from the last processed file in case of interruptions.
Ensure scalability for large datasets by introducing file sharding.
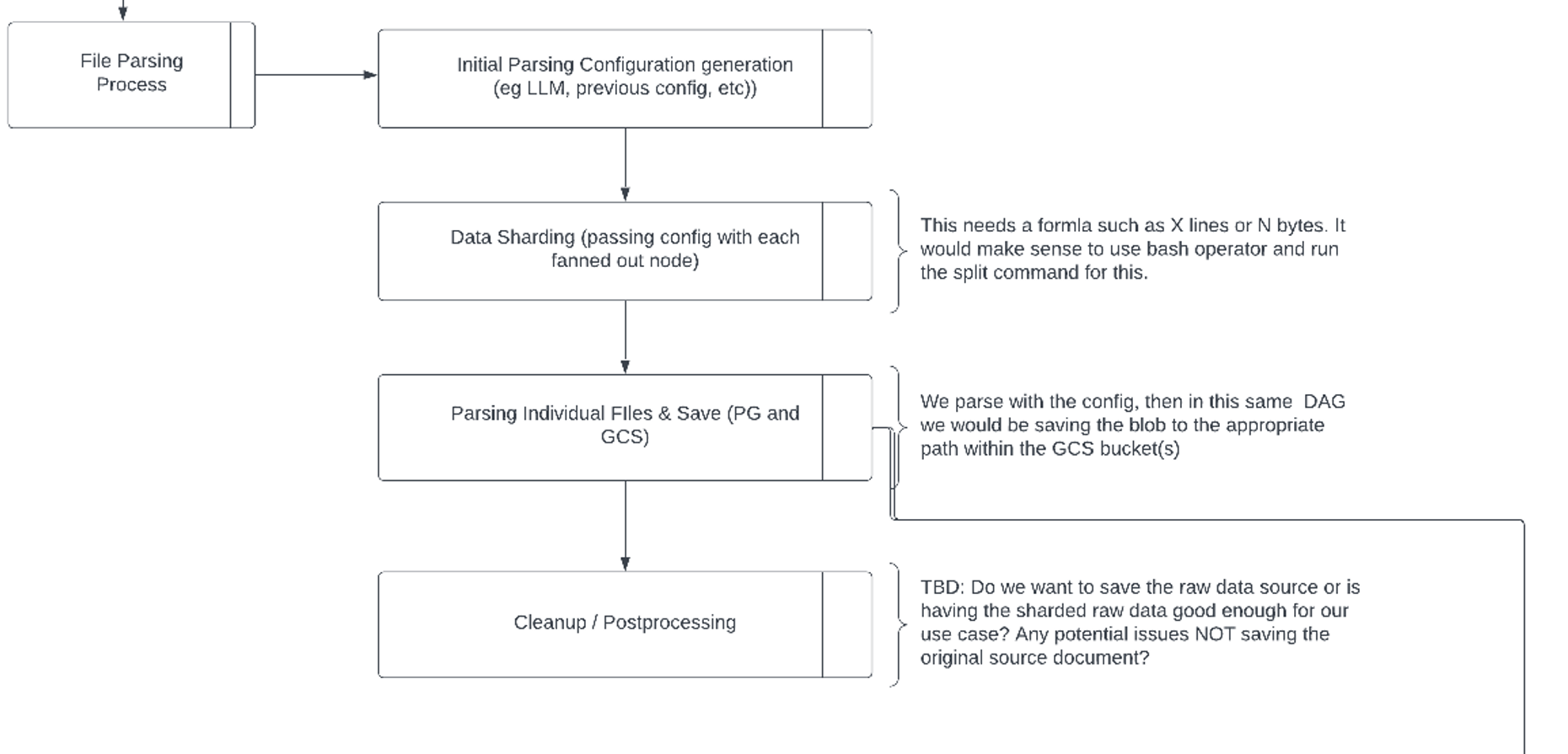
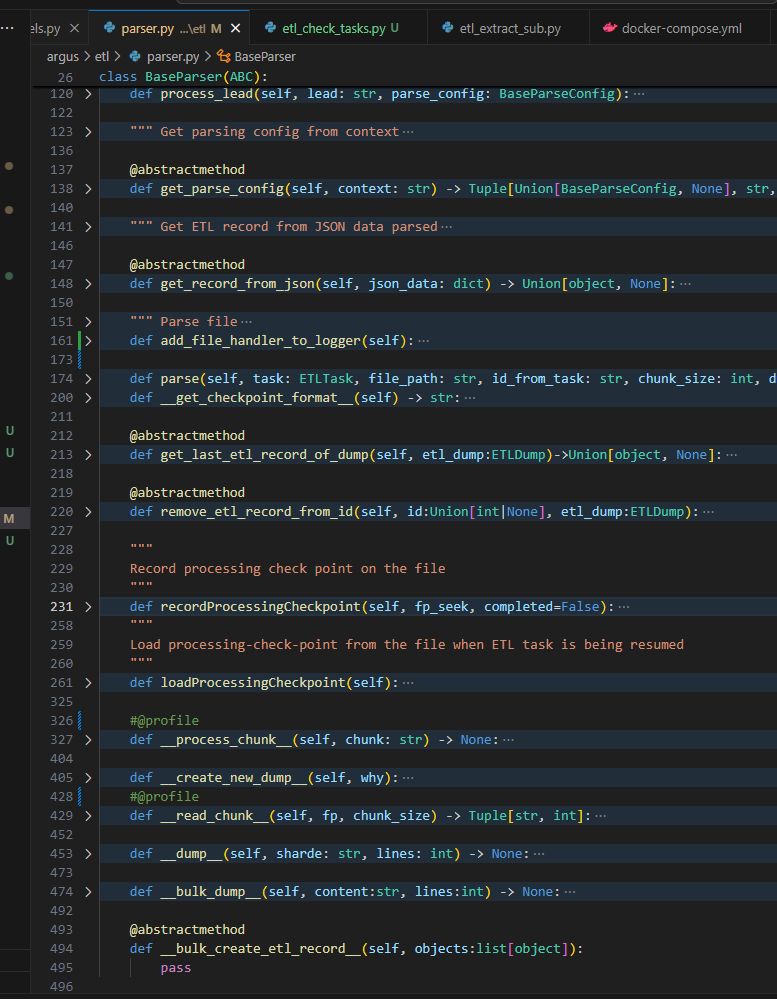
Solutions
Data Collection: Aggregate individual raw data files from the source location.
Dynamic Parsing Configuration: For each file, retrieve an existing parsing configuration or use Langchain to auto-generate one via LLM if it does not exist.
File Sharding for Large Files: Split large files into smaller shards to improve processing speed and reduce memory load.
Data Parsing and Storage: Apply parsing configurations to each shard, extracting structured data. Store the parsed data in PostgreSQL using Django ORM.
Checkpoint-based Resume/Pause Functionality: Implemented using Python to maintain a checkpoint log for each processed file, enabling the system to pause and resume from the last checkpoint.
Results
A scalable, automated solution capable of handling large datasets.
Improved fault tolerance and reduced downtime with resume/pause capabilities.
Successfully structured and stored profiles for efficient use in downstream applications.
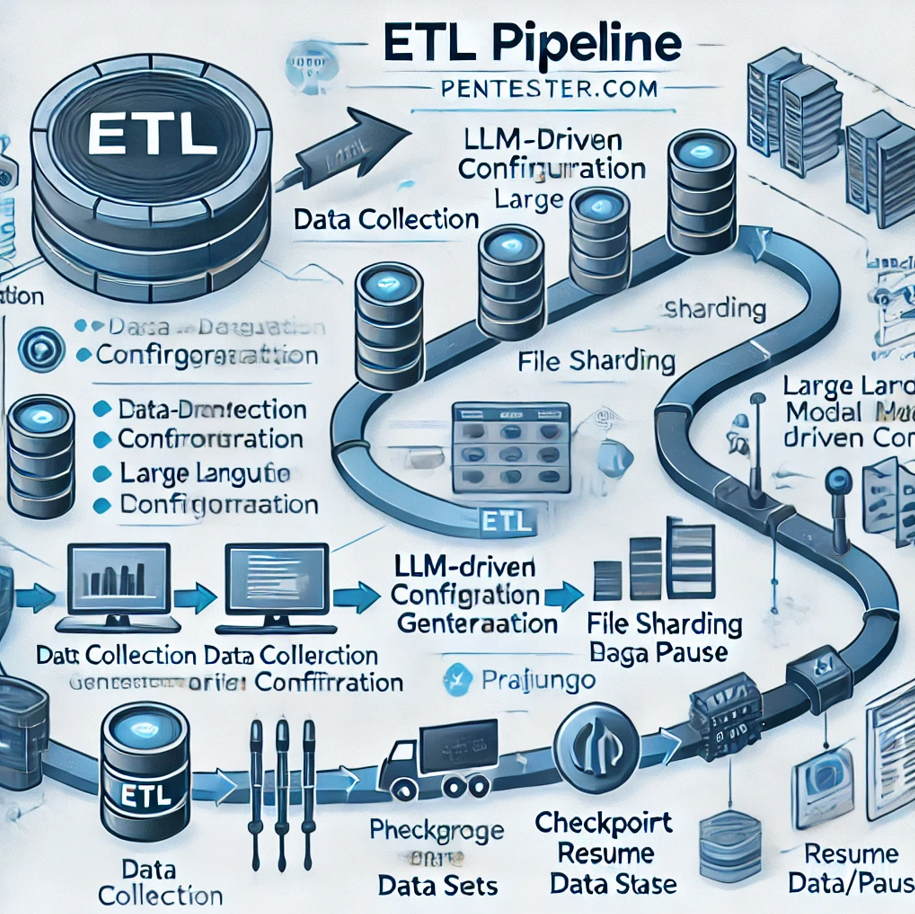